In this article
This is the first installment of a three-part interview series with Ahmad as we explore his hard-earned lessons as a supply chain leader at some of the best companies in the world.
During his time at Apple, he oversaw the launch and sustained delivery effort for tens of millions of iPad Minis.
In 2017, he joined the Allbirds team early in their journey and was instrumental in guiding the growth of their operations footprint from a small single-supplier system to one that could support hundreds of millions of dollars of annual sales.
Today, Ahmad is the VP of Operations at Firstbase, where they’re building an end-to-end platform that helps teams manage their global workforce. For him, this means figuring out how to get containers-worth of bulky furniture and expensive laptops all around the world in the fastest and cheapest manner, and back! On the side, he somehow finds time to advise operations-focused startups (like our team at Doss) and share his wealth of insights and tactical know-how.
**This conversation is being facilitated by Bala Pichumani, who covers a wide range of sales, marketing, and support for Team Doss.
What’s at the core of Apple’s operations?
Bala: What was the first time you were really in the weeds doing tactical work and thought like “Wow this is really hard”?
Ahmad: I was at Apple in their Supply and Demand Management team (think of this as a souped up S&OP team) and to be honest it was the best training ground for an operations person. That team at Apple’s HQ is global and central, and manages all the coordination between the supply and demand sides, as well as the various teams who managed other markets, factories, and components. This meant that there was a constant balancing act between the factories (the materials that Apple is buying and manufacturing) and linking it to the demand side, which is the forecast of sales that are happening on an ongoing basis across all channels and markets. SDM was basically a large optimization problem – with the info I have today, how can I best allocate available resources, both factory capacity and materials availability, to the right points-of-sale to maximize demand and minimize excess.
My first job there was launching the iPad Mini. So this was a while ago. And the work was launching this new product and needing to make sure that for this launch, all the countries in the world that will have this product have enough inventory to be able to be sold.
It required a lot of Excel modeling. I was just okay at Excel at that point in time, but everything at Apple in that team happened in Excel at that time. All the forecasting was in Excel. Everything from the inventory side was all Excel, there were no software systems in place except for pulling data which was quite extensive in terms of historical sales and information from the market teams around new sales activities. These were huge models to build and adjust to analyze decisions.
OK quick aside – this is a bit surprising, right? One of the most sophisticated companies in the world was launching their new products based on Excel demand models? Where was all of the custom forecasting software?
It turns out, that wasn’t really what they needed to get the job done – what they needed instead was a sophisticated group of operators to take models from just a “spreadsheet representing demand” and execute it into reality by constantly pushing this information to their suppliers, and being able to be reactive and flexible by using those same models to quickly turn around and take action.
Ahmad: Apple was very detailed in some ways. You had to forecast how much each SKU would sell, like this many black, with this storage amount, and this wireless carrier, in the US and this many of a different configuration in China, and so on…
You would use that to model the inventory when you actually go to manufacture it at which factory. And then you would use that to also tell all the teams who managed the parts vendors, like the Samsung’s of the world, “hey I need this many LCDs to be made to produce these many iPads.”
Again, a short interjection. If you’ve ever looked at any of the IFIXIT teardowns of Apple products you’re probably aware of just how many parts are in an iPad. This photo below is just one-side of their main processor printed circuit board and has dozens of distinct components spread across suppliers (including their own chip designs that are produced by TSMC). It’s not a stretch of the imagination to believe that these Excel models must have been massive.

Where this really got interesting for Ahmad was how the supply plans would change over time.
Ahmad: So this demand and supply plan that you would do every single week, maybe twice a week, but the details would change multiple times a day because assumptions would change. And you would have to kind of go to this Nth level of detail to share with factories through email and Excel file saying “Hey, go build this.” And we would call them at night saying “This is the model, this is the file, please go do exactly this.” Over time this changed and became more automated of course.
We also had to ensure that our customer commitments were being kept since Sales would use that to ensure that each POS was stocked and any change in supply would have reverberations down the supply chain. I think inherent in the excel modeling was the flexibility, that you could do exactly what you wanted vs. running it through a system. That flexibility was key to meeting customer commits.
We also built multiple scenarios of demand where we would do scenarios like a base case, a high case, a low case, etc. Each market, each country has its own forecast. If they came in a different percentage above what you had forecasted, what would you do? So if it came in higher, then your order that you already placed with the factory would run out very fast. Then it would take longer to resupply because your vendors had lead times to screens or batteries or enclosures, whatever. So everything had to be redone and re-forecast. So it all depends on the component that was in shortage.
And then on the flip side, if the demand came in a lot softer, now we had way too much supply. We had goals or targets of how much inventory we wanted to keep in a warehouse or how much inventory we wanted to keep on the boat due to long lead times or how much inventory we wanted to keep at a Best Buy. And those changed because our demand came in lower. Now we had to go negotiate with the Best Buy’s or Walmart’s saying, “Do you want more or less?” We’d go to our Sales team and say, “Hey, I have this much inventory, can you go push this?” All the decisions in the demand and supply side impacted the entire Apple framework.
This kind of end-to-end system of demand and supply is one that’s inextricably interconnected, from their sub-vendors all the way to their retail partners. What’s even more clear is that forecasting demand and supply wasn’t just a singular exercise that happened upfront. It was an ongoing activity that the whole business engaged in so that they could respond to a change in SKU-mix / geography-level demand all the way down to placing more orders with their component vendors or changing up their freight schedules.
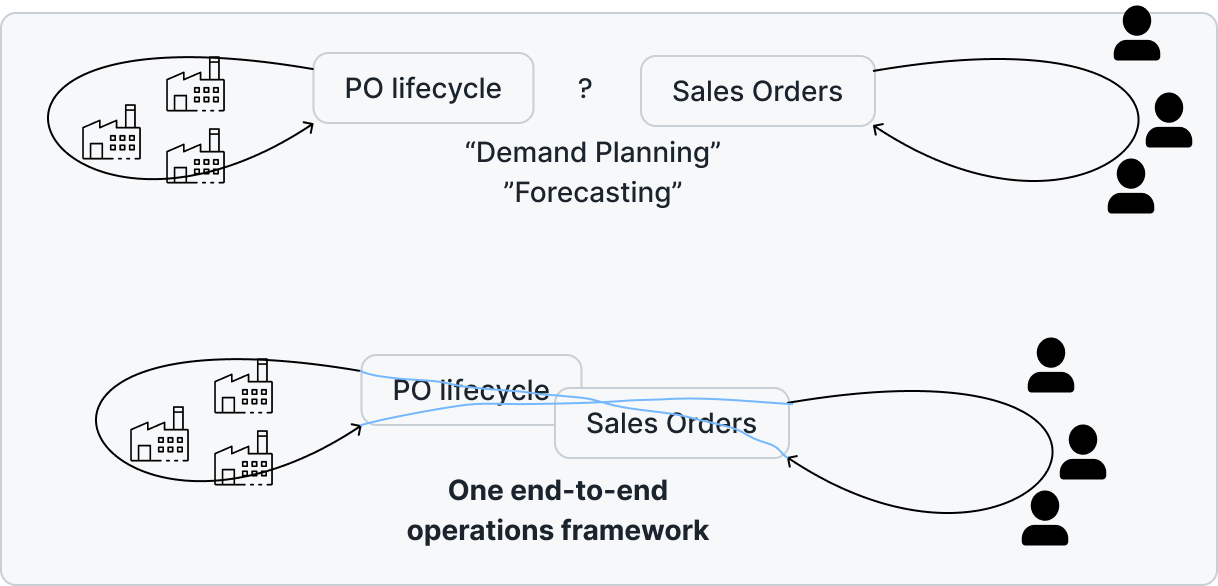
What’s particularly unique about Apple is the durability and sheer size of their demand. Not that many companies in the world need this level of hyper-specificity and responsiveness to market changes while also doing it all at such a massive scale. How does this pattern of One End-to-End Operations Framework translate outside of the domain of a $2T company?
Connecting the dots at Allbirds
Bala: What was different at a smaller scale like Allbirds, in a startup environment and an early-stage supply chain?
Ahmad: Certain supply chains require different things. If you're mostly ordering from a wholesaler in China, easy – it's already made. But if parts are manufactured from your own factory, you have multiple layers or tiers of materials. Our demand forecast needed to be translated into how many sheep were needed to farm in New Zealand, right? I'm making it a lot more sexy than it is, but it really was “Hey, we need to tell the farm how much wool we require on an annual basis.”
So that demand you apply to an early-stage supply chain can be completely wrong because you'd make a decision like a year or two years ahead. Then the question is how do you create alerts and close the feedback loop. Let’s say your demand is fluctuating a lot. Sales come in actually 50% below the forecasted target. You need to go cancel your orders. At the early stage, people don’t even have the data to connect the dots like “Hey, I ordered X quantity of this six months ago. My demand just crashed. I'm still on the hook for that. Can I go and cancel that order or reallocate the raw materials? What’s the impact on my working capital? When will I recover the cash I already spent on those commitments?"
On the surface it might not be clear if there's anything in common between Apple and Allbirds. Durable and predictable demand vs. sales volumes swinging 50% off from forecast. Ahmad thinks otherwise.
Ahmad: The question is what information is required, who needs it, who's doing the work of centralizing the key information, and who’s responsible for this all happening because it's very manual. So I think that's still a case that happens in Allbirds and even in Apple. It wasn't as bad, but it happened.
If you don't have a layer above a data warehouse, it's impossible to know what's going on. It’s very hard to know how a business is doing if it's all done manually. Somebody has to go pull reports from ten different places, consolidate it into another report and then clearly explain “This is what's happened” to a bunch of people.
Bala: Do you think all businesses that are scaling or growing quite rapidly and dealing with physical goods need this data layer?
Ahmad: That’s right. Again, if you are using tools that are new, like Shopify, you have part of it somewhere. There's an API that you can pull from.
But the issue is that not many companies or SaaS products have the rest of the operational data. Not many people have PO data. How many people have shipment data? SKU X was shipped on Date A, but SKU X was not shipped again on Date B. How much was it shipped? What was the price of it?
Companies will focus a lot on understanding demand, marketing data, ads data. They don’t focus on asset data or physical data.
His view is that it’s a short-sighted trade-off to only consider the demand side of the equation because the limits which levers you can pull as a business to address changes. More important than exactness in forecast accuracy is having operational data accuracy which powers real-time automation like low-stock alerts that trigger PO issuance.
Ahmad: I think for an operations team (the good ones) they need to be cognizant that you will always be wrong. Your forecast will always, 100% of the time, be wrong. It just depends on how much and in what direction.
How much do you trust that decision that you made? And you create some sort of flexibility and agility around that supply chain.
Think through the scenarios and tradeoffs. Find buffers where you don't impact either availability of inventory or cost of inventory. If demand is higher than you forecast then you're losing sales. But if demand is low then you bought stuff that you can't sell anymore.
Bala: So I guess the data accuracy unlocks flexibility to respond fast?
Ahmad: Data accuracy, the data portfolio (what pieces of data), and data refresh rate, right? Like how often are we getting this information now?
Bala: And so what's the ideal solution? 100% real time? 100% accurate?
Ahmad: Accuracy wouldn't be there. I think it's just having the tools to be able to make an informed decision and take action.
First, make sure most of the data is available. And then make it so that the data can be qualified, either by hand or some kind of trigger mechanism. Like I can figure out from context that this is a risk, or this triggers the automation of a manual task.
It’s also an issue of how you structure your teams. In very traditional apparel businesses, there's a Demand team. It's separate from the Supply team. Right, so they don't know what's going on with one another, they don't talk as much as they should. So they make different decisions.
To get everyone on the same page, both teams need to have the same information at all times. How do you build the right portals to the same information? How do you make sure that the person who's downstream is aware of changes in information? I would say data refresh is really about visibility and the awareness of change – not just time.
Adaptive by Design
At Doss, we agree with Ahmad’s vision for adaptive operations software – so much so that we named our platform after it: Doss Adaptive Resource Platform (ARP). But it’s more than just a clever acronym. Adaptive by Design is the guiding principle underpinning the entire platform. Every aspect of the data model and user experience empowers operators to customize, augment, overhaul, and adapt their workflows without friction.
Why? Because we know that change is inevitable. Every business is unique and growth compounds this uniqueness. Launching new products, adding new warehousing, onboarding new vendors. With Doss, you can go-live with tailored workflows faster than you could even get a quote from a traditional ERP vendor.
So, where does Doss fit into solving the key challenges Ahmad identified?
- Data Availability: Orchestrate and aggregate data across the end-to-end value-chain between your own data warehouses and 3rd-party systems
- Awareness: Create shared dashboards between cross-functional teams (Ops, Sales, Marketing, Supply Chain, etc) to give real-time visibility and granular detail
- Real-time Automation: Build Workflows in Doss that take your team from reactive to proactive. Automate Bulk PO creation, Wholesale Invoicing, Low-Stock Reordering, Order Delay Alerts, Shopify Data Syncs and so much more.

Get started today with our 30-day risk-free trial. No contract, no credit cards, and minimal lift on your part. Since Doss is so easy to configure, we don't charge anything to get you set up.
Prefer to see Doss in action? Book a demo today and start your journey with a platform that evolves with you.